Abstract
It is generally believed that a metaphor tends to have a stronger emotional impact than a literal statement; however, there is no quantitative study establishing the extent to which this is true. Further, the mechanisms through which metaphors convey emotions are not well understood. We present the first data-driven study comparing the emotionality of metaphorical expressions with that of their literal counterparts. Our results indicate that metaphorical usages are, on average, significantly more emotional than literal usages. We also show that this emotional content is not simply transferred from the source domain into the target, but rather is a result of meaning composition and interaction of the two domains in the metaphor.
1 Introduction
Metaphor gives our expression color, shape and character. Metaphorical language is a result of complex knowledge projection from one domain, typically a physical, closely experienced one, to another, typically more abstract and vague one (Lakoff and Johnson, 1980) . For instance, when we say "He shot down all of my arguments", we project knowledge and inferences from the domain of battle (the source domain) onto our reasoning about arguments and debates (the target domain). While preserving the core meaning of the sentence, the use of metaphor allows us to introduce additional connotations and emphasize certain aspects of the target domain, while downplaying others. Consider the following examples:
(1) a. The new measures are strangling business.
B. The New Measures Tightly Regulate Business.
When we talk about "strangling business" in (1a) we express a distinct viewpoint on governmental regulation of business, as opposed to a more neutral factual statement expressed in (1b).
The interplay of metaphor and emotion has been an object of interest in fields such as linguistics (Blanchette et al., 2001; Kovecses, 2003) , political science (Lakoff and Wehling, 2012) , cognitive psychology (Crawford, 2009; Thibodeau and Boroditsky, 2011) and neuroscience (Aziz-Zadeh and Damasio, 2008; Jabbi et al., 2008) . A number of computational approaches for sentiment polarity classification of metaphorical language have also been proposed (Veale and Li, 2012; Kozareva, 2013; Strzalkowski et al., 2014) . However, there is no quantitative study establishing the extent to which metaphorical language is used to express emotion nor a data-supported account of the mechanisms by which this happens.
Our study addresses two questions: (i) whether a metaphorical statement is likely to convey a stronger emotional content than its literal counterpart; and (ii) how this emotional content arises in the metaphor, i.e. whether it comes from the source domain, or from the target domain, or rather arises compositionally through interaction of the source and the target. To answer these questions, we conduct a series of experiments, in which human subjects are asked to judge metaphoricity and emotionality of a sentence in a range of settings. We test two experimental hypotheses.
Hypothesis 1: metaphorical uses of words tend to convey more emotion than their literal paraphrases in the same context.
Hypothesis 2: the metaphorical sense of a word tends to carry more emotion than the literal sense of the same word.
To test Hypothesis 1, we compare the emotional content of a metaphorically used word to that of its literal paraphrase in a fixed context, as in the following example.
(2) a. Hillary brushed off the accusations.
b. Hillary dismissed the accusations.
To test Hypothesis 2, we compare the emotional content of the metaphorical sense of a word to a literal sense of that same word in its literal context, as follows.
(3) a. Hillary brushed off the accusations. b. He brushed off the snow.
Here, brushed off is metaphorical in the context of "accusations" but literal in the context of "snow". Our experiments focus on metaphors expressed by a verb, since this is the most frequent type of metaphor, according to corpus studies (Cameron, 2003; Shutova and Teufel, 2010) . In order to obtain a sufficient coverage across metaphorical and literal verb senses we extract our data from Word-Net. For 1639 senses of 440 verbs, we annotate their usage as metaphorical or literal using the crowdsourcing platform, CrowdFlower 1 . We then create datasets of pairs of these usages to test Hypotheses 1 and 2.
Our results support both hypotheses, providing evidence that metaphor is an important mechanism for expressing emotions. Further, the fact that metaphorical uses of words tend to carry more emotion than their literal uses indicates that the emotional content is not simply transferred from the source domain into the target, but rather is a result of meaning composition and interaction of the two domains in the metaphor. For this project, we created a dataset in which verb senses are annotated for both metaphoricity and emotionality. In addition, the metaphorical uses are paired with their human-validated interpretations in the form of literal paraphrases. We have made this dataset freely available online. 2 We expect that this dataset, the first of its kind, will find many applications in NLP, including the development and testing of metaphor identification and interpretation systems, modeling regular polysemy in word sense disambiguation, distinguishing between near-synonyms in natural language generation, and, not least, the development of sentiment analysis systems that can operate on real-world, metaphor-rich texts.
1 www.crowdflower.com 2 http://saifmohammad.com/WebPages/metaphor.html
2 Related Work
Word sense, metaphor and emotion: The standard approach to word sense disambiguation (WSD) is to develop a model for each polysemous word (Navigli, 2009) . The model for a word predicts the intended sense, based on context. A problem with this approach to WSD is that good coverage of common polysemous English words would require about 3,200 distinct models. Kilgarriff (1997) has argued there are systematic relations among word senses across different words, focusing in particular on metaphor as a ubiquitous source of polysemy. This area of research is known as regular polysemy. Thus, there is a systematic relation between metaphor and word sense (Kilgarriff, 1997; Turney et al., 2011) and the emotion associated with a word depends on the sense of the word (Strapparava and Valitutti, 2004; Mohammad and Turney, 2013) . 3 This raises the question of whether there is a systematic relation between presence of metaphor and the emotional content of words. As far as we know, this is the first paper to quantitatively explore this question. Gibbs et al. (2002) conducted a study that looked at how listeners respond to metaphor and irony when they are played audio tapes describing emotional experiences. They found that on average metaphors were rated as being more emotional than non-metaphoric expressions. However, that work did not compare paraphrase pairs that differed in just one word (metaphorically or literally used) and thus did not control for context. Citron and Goldberg (2014) compared metaphorical and literal sentences differing only in one word, and found that metaphorical sentences led to more activity in the the amygdala and the anterior portion of the hippocampus. They hypothesized that this is because metaphorical sentences are more emotionally engaging than literal sentences.
Metaphor Annotation:
Metaphor annotation studies have typically been corpus-based and involved either continuous annotation of metaphorical language (i.e., distinguishing between literal and metaphorical uses of words in a given text), or search for instances of a specific metaphor in a corpus and an analysis thereof. The majority of corpus-linguistic studies were concerned with metaphorical expressions and mappings within a limited domain, e.g., WAR, BUSINESS, FOOD or PLANT metaphors (Santa Ana, 1999; Izwaini, 2003; Koller, 2004; Skorczynska Sznajder and Pique-Angordans, 2004; Lu and Ahrens, 2008; Low et al., 2010; Hardie et al., 2007) , in a particular genre or type of discourse (Charteris-Black, 2000; Cameron, 2003; Lu and Ahrens, 2008; Martin, 2006; Beigman Klebanov and Flor, 2013) .
Two recent studies (Steen et al., 2010; Shutova and Teufel, 2010) moved away from investigating particular domains to a more general study of how metaphor behaves in unrestricted continuous text. Steen and colleagues (Pragglejaz Group, 2007; Steen et al., 2010) proposed a metaphor identification procedure (MIP), in which every word is tagged as literal or metaphorical, based on whether it has a "more basic meaning" in other contexts than the current one. The basic meaning was defined as "more concrete; related to bodily action; more precise (as opposed to vague); historically older" and its identification was guided by dictionary definitions. Shutova and Teufel (2010) extended MIP to the identification of conceptual metaphors along with the linguistic ones. Lönneker (2004) investigated metaphor annotation in lexical resources. Their Hamburg Metaphor Database contains examples of metaphorical expressions in German and French, which are mapped to senses from Eu-roWordNet 4 and annotated with source-target domain mappings taken from the Master Metaphor List (Lakoff et al., 1991) .
Emotion annotation: Sentiment analysis is defined as detecting the evaluative or affective attitude in text. A vast majority of work in sentiment analysis has focused on developing classifiers for valence prediction (Kiritchenko et al., 2014; Dong et al., 2014; Socher et al., 2013; , i.e., determining whether a piece of text expresses positive, negative, or neutral attitude. However, there is a growing interest in detecting a wider range of emotions such as joy, sadness, optimism, etc. (Holzman and Pottenger, 2003; Alm et al., 2005; Brooks et al., 2013; Mohammad, 2012) . Much of the this work has been influenced by the idea that some emotions are more basic than others (Ekman, 1992; Ekman and Friesen, 2003; Plutchik, 1980; Plutchik, 1991) . Mohammad (2012) polled the Twitter API for tweets that have hashtag words such as #anger and #sadness corresponding to the eight Plutchik basic emo-tions. He showed that these hashtag words act as good labels for the rest of the tweets. Suttles and Ide (2013) used a similar distant supervision technique and collected tweets with emoticons, emoji, and hashtag words corresponding to the Plutchik emotions. Emotions have also been annotated in lexical resources such as the Affective Norms for English Words, the NRC Emotion Lexicon (Mohammad and Turney, 2013), and WordNet Affect (Strapparava and Valitutti, 2004) . The annotated corpora mentioned above have largely been used as training and test sets, and the lexicons have been used to provide features for emotion classification. (See Mohammad (2016) for a survey on affect datasets.) None of this work explicitly studied the interaction between metaphor and emotions.
3 Experimental Setup
To test Hypotheses 1 and 2, we extracted pairs of metaphorical and literal instances from Word-Net. In WordNet, each verb sense corresponds to a synset, which consists of a set of near-synonyms, a gloss (a brief definition), and one or more example sentences that show the usage of one or more of the near-synonyms. We will refer to each of these sentences as the verb-sense sentence, or just sentence. The portion of the sentence excluding the target verb will be called the context. We will refer to each pair of target verb and verb-sense sentence as an instance. We extracted the following types of instances from WordNet: Here, erase is used metaphorically. We will refer to such instances as metaphorical instances.
Now consider an instance similar to the one above, but where the target verb is replaced by its near-synonym or hypernym. For example: The sentence in Instance 2 has a different target verb (although with a very similar meaning to the first) and an identical context w.r.t. Instance 1. However, in this instance, the target verb is used literally. We will refer to such instances as literal instances. To test Hypothesis 1, we will compare pairs such as Instance 1-Instance 2. We will then ask human annotators to examine these instances both individually and in pairs to determine how much emotion the target verbs convey in the sentences.
Another instance of the verb erase, corresponding to a different sense, is shown below:
Instance 3
Target verb: erase Sentence: Erase the formula on the blackboard.
This instance contains a literal use of erase. To test Hypothesis 2, we will compare pairs such as Instance 1-Instance 3 that have the same target verb, but different contexts such that one instance is metaphorical and another is literal. We will ask human annotators to examine these instances both individually and in pairs to determine how much emotion the target verbs convey in the sentences.
In the sub-sections below, we describe: (3.1) How we compiled instance pairs to test Hypotheses 1 and 2. This involved annotating instances as metaphorical or literal. (3.2) How we annotated pairs of instances to determine which is more metaphorical. (33) How we annotated instances for emotionality. And finally, (3.4) how we annotated pairs of instances to determine which is more emotional.
3.1 Compiling Pairs Of Instances
In order to create datasets of pairs such as Instance 1-Instance 2 and Instance 1-Instance 3, we first determine whether WordNet verb instances are metaphorical or literal. Specifically, we chose verbs with at least three senses (so that there is a higher chance of at least one sense being metaphorical) and less than ten senses (to avoid highly ambiguous verbs). In total, 440 verbs satisfied this criterion, yielding 1639 instances. We took example sentences directly from WordNet and automatically checked to make sure that the verb appeared in the gloss and the example sentence. In cases where the example sentence did not contain the focus word, we ignored the synset. We used the Questionnaire 1 to annotate these instances for metaphoricity:
Questionnaire 1: Literal or Metaphorical? Instructions You will be given a focus word and a sentence that contains the focus word (highlighted in bold). You will be asked to rate whether the focus word is used in a literal sense or a metaphorical sense in that sentence. Below are some typical properties of metaphorical and literal senses:
Literal usages tend to be: -more basic, straightforward meaning; more physical, closely tied to our senses: vision, hearing, touching, tasting Metaphorical usages tend to be: -more complex; more distant from our senses; more abstract; more vague; often surprising; tend to bring in imagery from a different domain
Example 1
Focus Word: shoot down Sentence: The enemy shot down several of our aircraft.
Question: In the above sentence, is the focus word used in a literal sense or a metaphorical sense?
-the focus word's usage is metaphorical -the focus word's usage is literal Solution: the focus word's usage is literal
Example 2
Focus Word: shoot down Sentence: He shot down the student's proposal.
Question: In the above sentence, is the focus word used in a literal sense or a metaphorical sense?
-the focus word's usage is metaphorical -the focus word's usage is literal Solution: the focus word's usage is metaphorical
Your Task
Focus Word: answer Sentence: This steering wheel answers to the slightest touch.
In the above sentence, is the focus word used in a literal sense or a metaphorical sense? -the focus word's usage is metaphorical -the focus word's usage is literal This questionnaire, and all of the others described ahead in this paper, were annotated through the crowdsourcing platform Crowd-Flower. The instances in all of these questionnaires were presented in random order. Each instance was annotated by at least ten annotators. CrowdFlower chooses the majority response as the answer to each question. For our experiments, we chose a stronger criterion for an instance to be considered metaphorical or literal -70% or more of the annotators had to agree on the choice of the category. The instances for which this level of agreement was not reached were discarded from further analysis. This strict criterion was chosen so that greater confidence can be placed on the results obtained from the annotations. Nonetheless, we release the full set of 1,639 annotated instances for other uses and further research. Additionally, we selected only those instances whose focus verbs had at least one metaphorical sense (or instance) and at least one literal sense (or instance). This resulted in a Master Set of 176 metaphorical instances and 284 literal instances.
Focus Word 1: attack Sentence 1: I attacked the problem as soon as I was up. Focus word 2: attack Sentence 2: The Serbs attacked the village at night.
Which is more metaphorical? -focus word's usage in the sentence 1 is more metaphorical -the focus word's usage in sentence 2 is more metaphorical -the usages in the two sentences are equally metaphorical or equally literal
The instance pairs within a question were presented in random order. The questions themselves were also in random order.
Focus Word: answer Sentence: This steering wheel answers to the slightest touch.
How much emotion is conveyed? -the focus word conveys some emotion -the focus word conveys no emotion
Focus Word 1: attack Sentence 1: I attacked the problem as soon as I was up. Focus word 2: start Sentence 2: I started on the problem as soon as I was up.
Which conveys more emotion? -focus word in first sentence conveys more emotion -focus word in second sentence conveys more emotion -focus words in the two sentences convey a similar degree of emotion
The order in which the instance pairs were presented for annotation was determined by random selection. Whether the metaphorical or the literal instance of a pair was chosen as the first instance shown in the question was also determined by random selection. The same questionnaire was used for Hypothesis 2 pairs as well.
3.1.1 Instances To Test Hypothesis 1
For each of the 176 metaphorical instances in the Master Set, the three authors of this paper independently chose a synonym of the target verb that would make the instance literal. For example, for Instance 1 shown earlier, kill was chosen as synonym of erase (forming Instance 2). The synonym was chosen either from the list of near-synonyms in the same synset as the target word or from WordNet hypernyms of the target word. The three authors discussed amongst themselves to resolve disagreements. Five instances were discarded because of lack of agreement. Thus corresponding to each of the remaining 171 metaphorical instances, 171 literal instances were generated that had nonidentical, similar meaning target verbs, but identical contexts. This set of 171 pairs of instances forms the dataset used to test Hypothesis 1, and we will refer to these instance pairs as the Hypothesis 1 Pairs and to the set of 342 (171×2) instances as the Hypothesis 1 Instances.
3.1.2 Instances To Test Hypothesis 2
In order to test Hypothesis 2, we compare instances with the same target verb, but corresponding to its different senses. We use all of the 460 (176+284) instances in the Master Set, and refer to them as Hypothesis 2 Instances. As for Hypothesis 1, we also group these instances into pairs. For each of the verbs in the Master Set, all possible pairs of metaphorical and literal instances were generated. For example, if a verb had two metaphorical instances and three literal instances, then 2 × 3 = 6 pairs of instances were generated. In total, 355 pairs of instances were generated. We will refer to his set of instance pairs as Hypothesis 2 Cross Pairs (pairs in which one instance is labeled metaphoric and the other is literal).
Rather than viewing instances as either metaphorical or literal, one may also consider a graded notion of metaphoricity. That is, on a scale from most literal to most metaphorical, instances may have different degrees of metaphoricity (or literalness). Therefore, we also evaluate pairs where the individual instances have not been explicitly labeled as metaphorical or literal; instead, they have been marked according to whether one instance is more metaphorical than the other. For each of the verbs in the Master Set, all possible pairs of instances were generated. For example, if a verb had five instances in the Master Set, then ten pairs of instances were generated. This resulted in 629 pairs in total. We will refer to them as Hypothesis 2 All Pairs (all possible pairs of instances, without regard to their labels).
3.2 Relative Metaphoricity Annotation
For each of the pairs in both Hypothesis 2 Cross Pairs and in Hypothesis 2 All Pairs, we ask annotators which instance is more metaphorical, as shown in Questionnaire 2 below:
Questionnaire 2: Which is more metaphorical? Instructions You will be given two sentences with similar meanings. Each sentence contains a focus word. You will be asked to compare how the focus words are used in the two sentences. You will be asked to decide whether the focus word's usage in one sentence is more metaphorical than the focus word's usage in the other sentence.
-Description of metaphorical and literal usages same as in Questionnaire 1 (not repeated here due to space constraints)-
3.3 Absolute Emotion Annotation
For each of the Hypothesis 1 and Hypothesis 2 instances, we used responses to Questionnaire 3 shown below to determine if the target verb conveys an emotion in the sentence.
Questionnaire 3: Does the focus word convey emotion? Instructions You will be given a focus word and a sentence that includes the focus word. You will be asked to rate whether the focus word conveys some emotion in the sentence.
3.4 Relative Emotion Annotation
Just as instances can have degrees of metaphoricity, they can have degrees of emotion. Thus, for each of the Hypothesis 1 Pairs we asked annotators to mark which instance is more emotional, as shown in Questionnaire 4 below:
Questionnaire 4: Which of the two given sentences conveys more emotion? Instructions You will be given two sentences with similar meanings. Each sentence contains a focus word. You will be asked to compare how the focus words are used in the two sentences and whether the focus word conveys more emotion in one sentence than in the other sentence.
4 Results And Data Analysis 4.1 Hypothesis 1 Results
An analysis of the responses to Questionnaire 3 for the Hypothesis 1 instances is shown in Table 1. Recall that the annotators were given 342 instances where half were metaphoric and half were literal. Additionally each literal instance was created by replacing the target verb in a metaphorical instance with a synonym of the target verb. Recall also that the 342 instances were presented in random order. Table 1 shows that a markedly higher number of metaphorical instances (39.8%) are considered emotional than literal ones (16.1%).
Fisher's exact test shows that this difference is significant with greater than 95% confidence 5 . An analysis of the responses to Questionnaire 4 for the Hypothesis 1 pairs is shown in Table 2 . Here, the annotators were given pairs of instances where one is metaphorical and one is literal (and the two instances differ only in the target verb), and the annotators were asked to determine which instance is more emotional. Metaphorical instances were again predominantly marked as more emotional (83.6%) than their literal counterparts (9.9%). This difference is significant with greater than 95% confidence, using the binomial exact test. Thus, results from both experiments support Hypothesis 1. Table 3 shows an analysis of the responses to Questionnaire 3 for the Hypothesis 2 instances. Recall that the annotators were given 460 instances where 176 were metaphoric and 284 were literal. The data corresponds to verbs that have both metaphorical and literal senses. The various instances generated for each verb have the same focus verb but different context (verb-sense sentence). The 460 instances were again presented in random order. Table 3 shows that a markedly higher number of metaphorical instances are considered emotional (14.1%), whereas much fewer of the literal instances are considered emotional (3.7%). This difference is significant with greater than 95% confidence, using Fisher's exact test. Hypothesis 2 All Pairs received lower overall emotionality scores than Hypothesis 1 Pairs. Some variation is expected because the two datasets are not identical. Additionally, when an annotator finds the same word in many literal (non-emotional contexts) as in the Hypothesis 2 setup (but not in Hypothesis 1 setup), then they are less likely to tell us that the same word, even though now used in a metaphorical context, is conveying emotion. Despite the lower overall emotionality of Hypothesis 2 All Pairs, our hypothesis that metaphorical instances are more emotional than the literal ones still holds. Further, experiments with pairs of emotions (described below) avoid the kind of bias mentioned above, and also demonstrate the higher relative emotionality of metaphorical instances. Table 4 shows the analysis for Hypothesis 2 Cross Pairs in the relative emotion annotation setting. The annotators were given pairs of instances where one is metaphorical and one is literal (and the two instances have the same focus verb in different context). The annotators were asked to determine which instance is more emotional. Metaphorical instances were marked as being more emotional than their literal counterparts in 59.4% of cases. Literal instances were marked as more emotional only in 8.7% of cases. This difference is significant with greater than 95% confidence, using the binomial exact test.
4.2 Hypothesis 2 Results
An analysis of the responses to Questionnaire 4 for the Hypothesis 2 All Pairs is shown in Table 5. This dataset included all possible pairs of instances associated with each verb in the Master Set. Thus in addition to pairs where one is highly metaphorical and one highly literal, this set also includes pairs where both instances may be highly metaphorical or both highly literal. Observe that (Table 4) : Life in the camp drained him. MET The rain water drains into this big vat. LIT -the first sentence conveys more emotion We drained the oil tank. LIT The exercise class drains me of energy. MET -the second sentence conveys more emotion Figure 1 : Complete annotation cycle for the verb drain (some sense pairs are omitted for brevity). LIT stands for literal and MET for metaphoric. The annotations in Q1 are accompanied by their confidence scores.
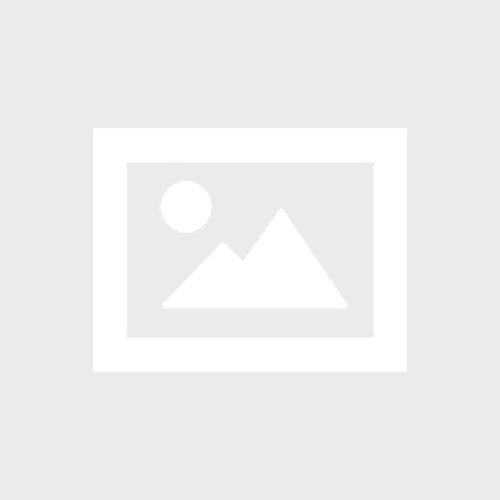
once again a higher number of instances that were marked as more metaphorical were also marked as being more emotional (than less or similarly emotional). This difference is significant with greater than 95% confidence (binomial exact test).
Overall, these results support Hypothesis 2, that metaphorical senses of the same word tend to carry more emotion than its literal senses. Figure 1 demonstrates the complete annotation cycle (Q1 to Q4) for the verb drain. Table 5 : Summary of responses to Q4 (which is more emotional) for Hypothesis 2 All Pairs (629 pairs of instances). Note that in addition to pairs where one is highly metaphorical and one highly literal, the All Pairs set also includes pairs where both instances may be highly metaphorical or both highly literal.
5 Discussion
It is generally believed that the senses of a word can be divided into a metaphorical subset and a literal subset (Kilgarriff, 1997) . It is easy to find examples of this particular pattern of polysemy, but a few examples do not justify the claim that this pattern is a widespread regularity. The annotations of our dataset confirm the hypothesis that the metaphorical/literal distinction is a common pattern for polysemous verbs (as many as 38% of all verb senses we annotated were metaphorical). As far as we know, this is the first study that gives a solid empirical foundation to the belief that the metaphorical/literal distinction is a central form of regular polysemy. Furthermore, the annotated dataset can be used for research into the nature of metaphorical/literal regular polysemy. Previous research on metaphor annotation identified metaphorical uses of words in text, thus analysing data for only one sense at a time. In contrast, our dataset allows one to analyse a range of metaphorical and literal uses of the same word, potentially shedding light on the origins of regular polysemy and metaphor. Such a structure of the dataset also provides a new framework for computational modelling of metaphor. A system able to systematically capture metaphorical sense extensions will be in a better position to generalise to unseen metaphors rather than a system trained on individual examples of metaphorical word uses in their specific contexts. The large size and coverage across many senses makes this dataset particularly attractive for computational modeling of metaphor. Our analysis also suggests that the work on emotion detection in text may be useful to support algorithms for handling metaphorical sense extension. Perhaps emotion analysis may yield insights into other forms of regular polysemy (Boleda et al., 2012) .
We hypothesized that literal paraphrases tend to express less emotion than their metaphorical coun-terparts. This conjecture is related to Hypothesis 1. All of the sentence pairs that we used to test Hypothesis 1 are essentially a special type of paraphrase, in which only one word is varied. The results in Section 4.1 support Hypothesis 1, and thus they lend some degree of support to our hypothesis about paraphrases. It might be argued that we have only tested a special case of paraphrase, and we agree that further experiments are needed, with more general types of paraphrase (including, for instance, multi-word paraphrases). We leave this as a topic for future work. However, our results confirm the validity of our hypothesis with respect to metaphorical and literal lexical substitutes.
The results of our experiments are also relevant to many other NLP tasks modelling lexical meaning, for instance, natural language generation (NLG). It can be difficult to make the correct choice among several near-synonyms in NLG (Inkpen and Hirst, 2006) ; for example, the nearsynonyms error, mistake, slip, and blunder convey the same core meaning but have different connotations. The degree to which two words are near-synonyms is proportional to the degree to which one can substitute for another in a given context (Inkpen and Hirst, 2006) . Substituting a metaphoric term with a literal one tends to change the meaning of the sentence in an important respect-its emotional content. The degree of metaphor in the generated sentences would thus become an important factor in selecting the most appropriate candidate in NLG. It follows from Hypothesis 1 that terms with the same degree of metaphor will be more substitutable than terms with different degrees of metaphor. Therefore NLG systems can benefit from taking the degree of metaphor into account.
Our experiments and data also provide new insights into the nature of metaphorical emotions. Our results confirm both hypotheses, supporting the claim that metaphorical uses of words carry stronger emotions than their literal uses, as well as their literal paraphrases. This suggests that emotional content is not merely a property of the source or the target domain (and the respective word senses), but rather it arises through metaphorical composition. Figure 2 shows some examples of this phenomenon in our dataset. This is the first such finding, and it highlights the importance of metaphor as a mechanism for expressing emotion. This, in turn, suggests that joint models of metaphor and emotion are needed in order to create real-world systems for metaphor interpretation, as well as for sentiment analysis. All of the data created as part of this project, as summarized in Table 6 , is made freely available. 6
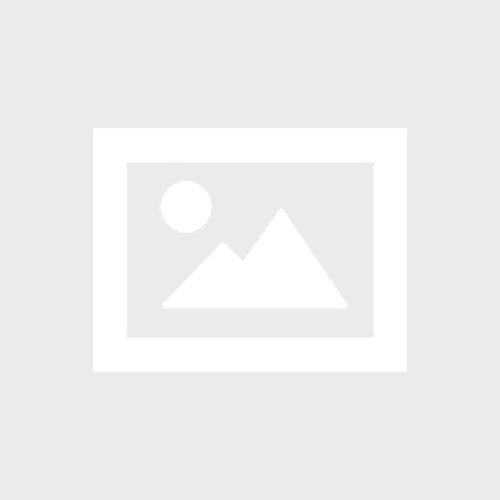
6 Conclusions
This paper confirms the general belief that metaphorical language tends to have a stronger emotional impact than literal language. As far as we know, our study is the first attempt to clearly formulate and test this belief. We formulated two hypotheses regarding emotionality of metaphors. Hypothesis 1: metaphorical uses of words tend to 6 http://saifmohammad.com/WebPages/metaphor.html convey more emotion than their literal paraphrases in the same context. Hypothesis 2: the metaphorical sense of a word tends to carry more emotion than the literal sense of the same word. We conducted systematic experiments to show that both hypotheses are true for verb metaphors. A further contribution of this work is to the areas of sentiment analysis and metaphor detection. At training time, sentiment classifiers could, for example, use the information that a particular word or expression is metaphorical as a feature, and similarly, metaphor detection systems could use the information that a particular word or expression conveys sentiment as a feature. The results are significant for the study of regular polysemy as the senses of many verbs readily divide into literal and metaphorical groups. We hope that research in regular polysemy will be able to build on the datasets that we have released. Our results also support the idea that a metaphor conveys emotion that goes beyond the source and target domains taken separately. The act of bridging the two domains creates something new, beyond the component domains. This remains a rich topic for further research. Finally, we hope that the results in this paper will encourage greater collaboration between the Natural Language Processing research communities in sentiment analysis and metaphor analysis. All of the data annotated for metaphoricity and emotionality is made freely available.
Words used in different senses convey different affect.
http://www.illc.uva.nl/EuroWordNet/
In the following experiments, we use Fisher's exact test for two-by-two tables of event counts and we use the binomial exact test (i.e., the Clopper-Pearson interval) for binary (heads/tails) event counts(Agresti, 1996).